Workshop Description
Proteins are one of the major workhorses of the human cell, and carry out major functions that keep us healthy. Many human genetic diseases, such as cystic fibrosis (CF) or Duchenne muscular dystrophy, are the result of mutations to proteins produced by the cells in our body. CF for example, involve mutations to a protein responsible for transporting ions across the membranes of our cell. Shown below is a visualization of the 3D structure of the CFTR gene that is mutated in cystic fibrosis patients, as well as a cartoon showing how the protein malfunctions in cystic fibrosis patients compared to healthy individuals (figure adapted from here).
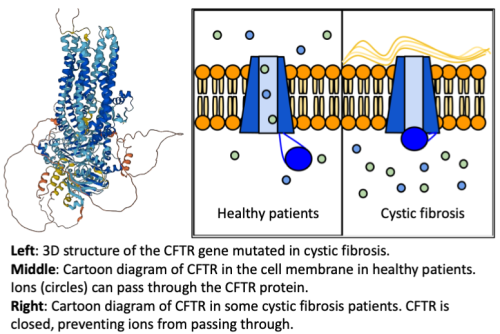
Seeing the protein 3D structure with and without a disease mutation is important for figuring out how the disease works, and how to develop therapies for it.
Genetic studies have identified many of these protein mutations that contribute to disease development, but unlike cystic fibrosis, we do not have a reliable way to predict what the effect of the mutation is on the protein structure, thus hindering efforts to develop therapies for these diseases. In the past few years, deep learning and other machine learning tools have been developed to allow us to accurate predict protein structure from its sequence, and hypothesize about how genetic mutations affect how our cells and bodies work. Below is an outline of how the deep learning tool AlphaFold2 works (adapted from here).
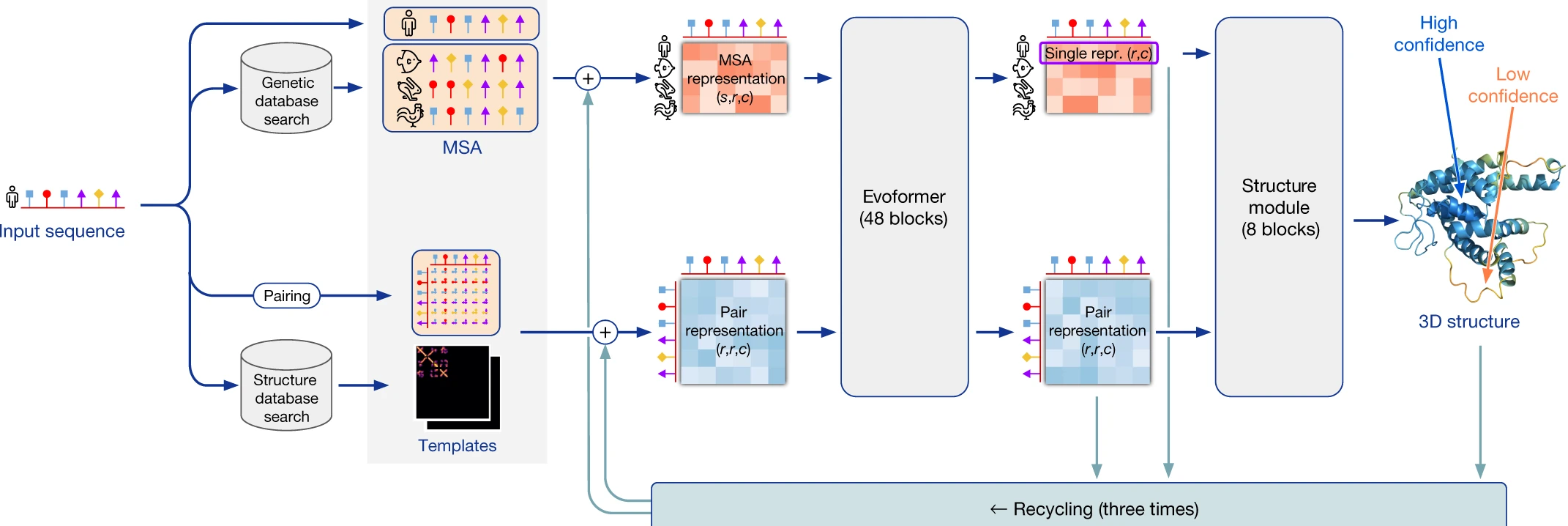
In this hands-on, interactive workshop, students will use deep learning tools such as AlphaFold2 to investigate the potential effects of protein coding mutations that are thought to drive different human diseases. Students will contribute to real-life research while also learning about how AI can be used in biology and medicine, gain practical experience using deep learning tools for making predictions, visualize 3D structures of proteins, interpret a scientific paper, visualize genomic data, and present research results.
No prior background or experience in biology or computer science is necessary for this workshop; all students are invited to apply.
Topics covered
Students will be assembled into teams of pairs of students for collaboration. Through these workshop activities, students will receive explicit training on interdisciplinary teamwork and presentation skills and will have opportunities to practice these skills through hands-on, collaborative work and an end-of-workshop presentation on their results. This is a hands-on lab; through practice and discussion, we will cover the following topics:
- What is a genome? Why are proteins important and how do they function? How do some genetic diseases work? Why do we need AI?
- How do we find out which diseases are likely caused by mutations in amino acid sequences of proteins? How do we find important parts of proteins, through analysis of protein sequences?
- How can we use deep learning to predict the 3D structure of proteins? How do we visualize it?
- How do we assess the quality of predictions made by deep learning?
- What is the effect of disease-causing mutations on the protein 3D structure?
- Can we use deep learning to predict which parts of the proteins are more important than others?
- How do we effectively communicate our results through formal scientific reports? Through oral presentations?
Educational Outcomes
(A) Students will actively participate in a research project that is broadly relevant to the academic community.
(B) Students will practice collaboration, iteration, creativity, and failure, through the tasks and assignments associated with the course.
(C) Students will gain practical understanding of how AI is used in biology and medicine applications.
(D) Students will gain experience diagnosing when (and when not) to believe deep learning predictions in the context of biology.
(E) Students will more strongly identify as researchers.
Time commitment
Workshops are typically one hour of live, hands-on interactive work and, on average, one hour of outside work per week, for ~4-6 months.